OBJECTIVE
Rayo Vallecano is a club that in recent years has been characterised by doing a good job on the pitch and also in the offices, forming and giving continuity to a group of players who have given a great performance, but at the end of this season, a large number of them will leave the team and the club will have to undertake a deep remodelling of the squad.
Since Rayo Vallecano achieved promotion to the first division in the 2020-2021 season, the team has not struggled to maintain the category, and has even been in the European places at times.
There are many factors that have led to these good results, such as the management of the until recently coach, Andoni Iraola, or also the continuity over time of great players who have formed the backbone of the team such as Óscar Trejo, Stole Dimitrievski, Alejandro Catena, Fran García, Isi Palazón, Óscar Valentín, Álvaro García or Santi Comesaña, players who were already in the team in the second division.
The confirmed departure of some of these players, such as Fran García to his club of origin, Real Madrid, Alejandro Catena to Atlético Osasuna, Florian Lejeune, who returns to Deportivo Alavés after finishing his loan or Santi Comesaña to Villarreal, leaves an important gap in the squad.
In addition to these losses, Sergio Camello also leaves the team, another player who, without the label of unquestionable starter, has had a very important impact on the team, fighting for the starting place with Raúl de Tomás, Rayo’s big bet up front.
Then we have the case of Radamel Falcao, a player whose departure was the end of the season, but nowadays it is not so clear.
Given this uncertainty and given that it is always good to have players of the so-called “specialists” in the squad, who can help in certain matches and at certain times, this study will also look for a player who could replace him when the time comes.
The “problem” with doing so well is that many teams with bigger budgets and squads designed to always play in European tournaments set their sights on these teams and they see opportunities to get established, high-level players at a low price or even worse, in some cases, directly at zero cost.
This project aims, through the use of data and Machine Learning, to explore the market of the five major leagues and other minor leagues to obtain a sample of players as similar as possible to those leaving the squad.
The structure of a squad can be formed based on many factors, it may not be necessary to replace all players leaving on a position by position basis, but these considerations must be carried out internally and, due to this circumstance, this study will be based on player by player, role by role replacement.
Once the selection of the sample has been made and the aspects that we consider relevant have been analysed, three main candidates1 will be given for each player to be replaced based on their skills, but also being consequent with the economic reality of the club.
This is a very important aspect to consider, the final selection of the sample will be based on Machine Learning, but then we will have to discard candidates that, objectively speaking, are unfeasible for the club.
As I don’t know the club’s budget limit, I have set a maximum market value price (based on Transfermarkt) of €8 million, which was the maximum price Rayo Vallecano paid for a player last season (Raúl de Tomás).

Source: Own elaboration.
1 It is possible that some of the players named in this report have changed team during the course of this project.
DATA AND SOURCES USED
All data used in this report is taken from the fbref website, and is up to date with all matchdays of the 2022-2023 season of the championships we have selected.
To carry out this tracking task it is important to have the data of the five big leagues (La Liga, Premier League, Serie A, Ligue 1 and Bundesliga), as being the most powerful, we can assume that it will be an advantage to get a consolidated player in one of them, But we have to consider that the salaries and market values of the players that play in these leagues make it very difficult for teams like Rayo Vallecano to access them, and that’s why I wanted to enrich the sample of players by adding another five leagues to the study.
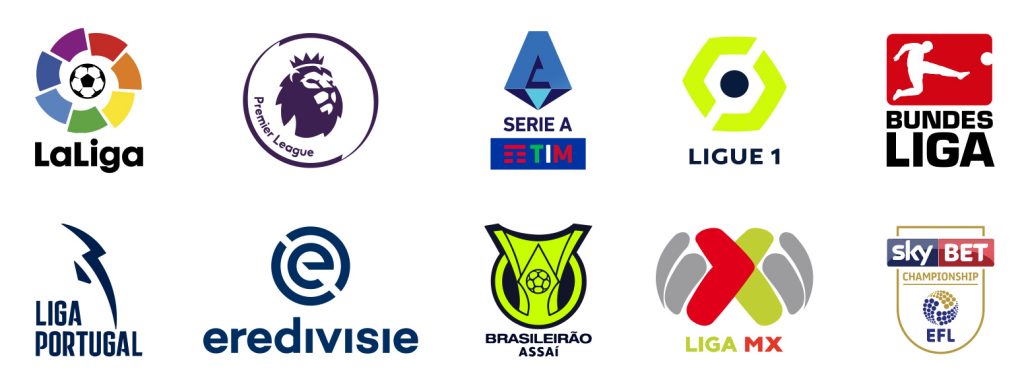
Each of these new leagues have their own characteristics that make them interesting to consider, for example, the Portuguese Primeira Liga is full of young talent (often coming from Brazil, as having the same language makes it the ideal place to make the leap to Europe for many untapped players) and veteran players who still have a few years at a high level; the Dutch Eredivisie has always been characterised by a lively and attractive game, similar to the one played in our country, as well as being a league that is very much committed to young talent; The Brazilian Serie A is the cradle of the great Brazilian players who later come to Europe; the Mexican Liga MX is a league that has evolved a lot in recent years and has experienced players and, on many occasions, players from the Argentinian and Uruguayan leagues; and finally, the Championship, the English second division, is a tremendously complicated league, I would say more powerful than some minor leagues in Europe.
I would like to have had the data of the Spanish and French second division, (championships with a great variety of very experienced players and with young players with a great margin of progression) but it has not been possible because fbref does not have the same amount of information as the selected leagues and it would not be adequate to evaluate players without having the same information for all of them.

ANALYTICAL MODELS
Once we have all our files prepared, we will apply Machine Learning using dimensionality reduction by means of the PCA (Principal Component Analysis) technique to obtain correlations between the players in the sample. To further refine the choice, we will apply the clustering technique and finish by performing a scoring that will help us to define our choice.

Dimensionality Reduction (PCA)
The problem with having a large amount of information is that we have numerous features that result in over-fitting.
To solve this, dimensionality reduction will be applied, which is basically the process of reducing the number of variables in the dataset by obtaining a set of main variables.
Put in a simpler way, what we want is to compress the dataset to eliminate redundant information and then, with this compressed dataset containing the metrics with the correct weights, we can extract correlations between all the players.
This process of applying dimensionality reduction and searching for the correlation of the players will be carried out entirely with Python.

Correlation results for all players

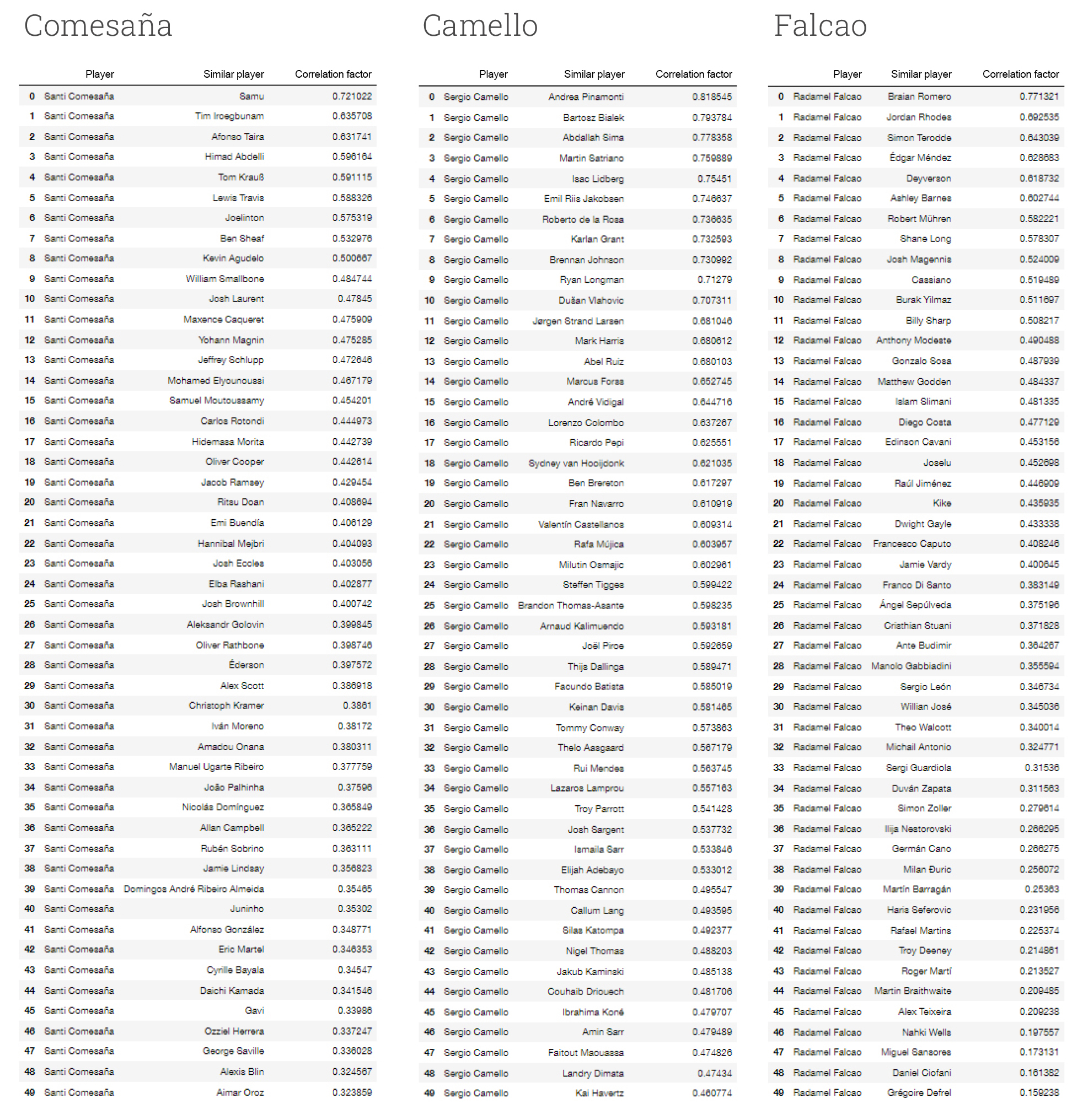
Clustering
Thanks to the process carried out with dimensionality reduction and similarity, we have managed to have a sample of similar players that will help us make a decision on which players may be the most suitable, but at this point, we are going to try to refine a little more.
We are going to try, from the final sample and considering that all these players are already very similar, to do a clustering to see which players would be closest to the player to be replaced.
Doing this clustering does not mean that we have to discard players who are not in the same group as the player to be replaced, it is just to have even more arguments to make the final decision.
Clustering results of all players
Alejandro Catena
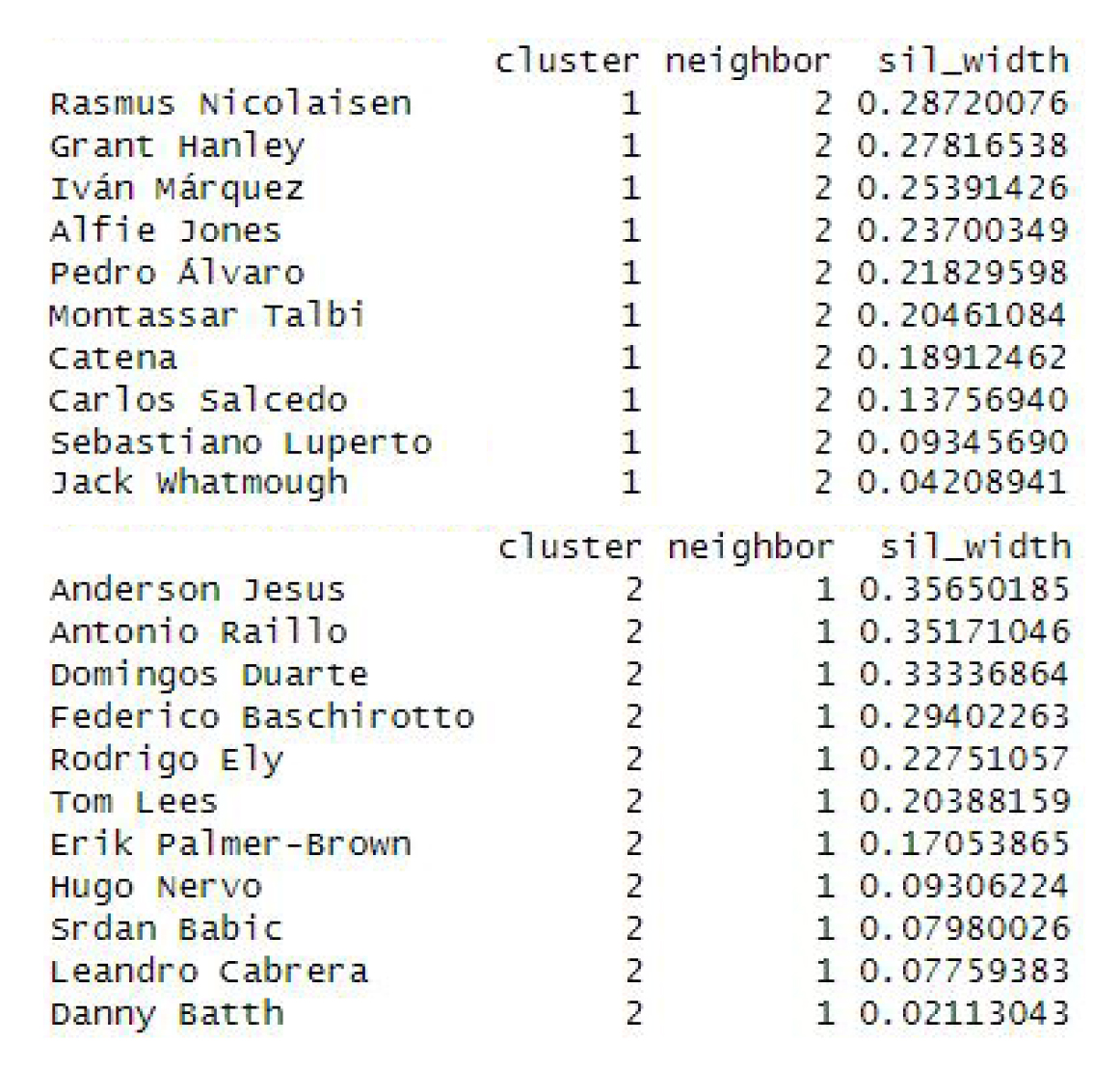
Florian Lejeune
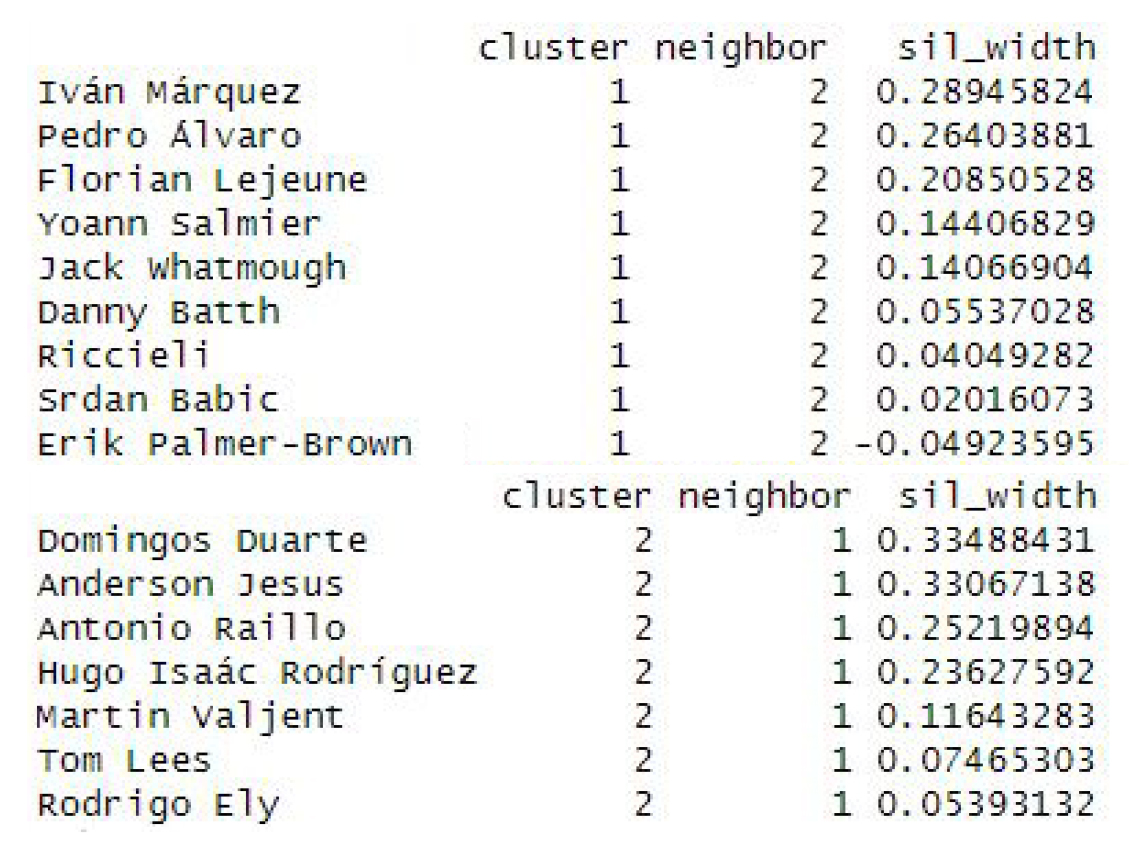
Fran García
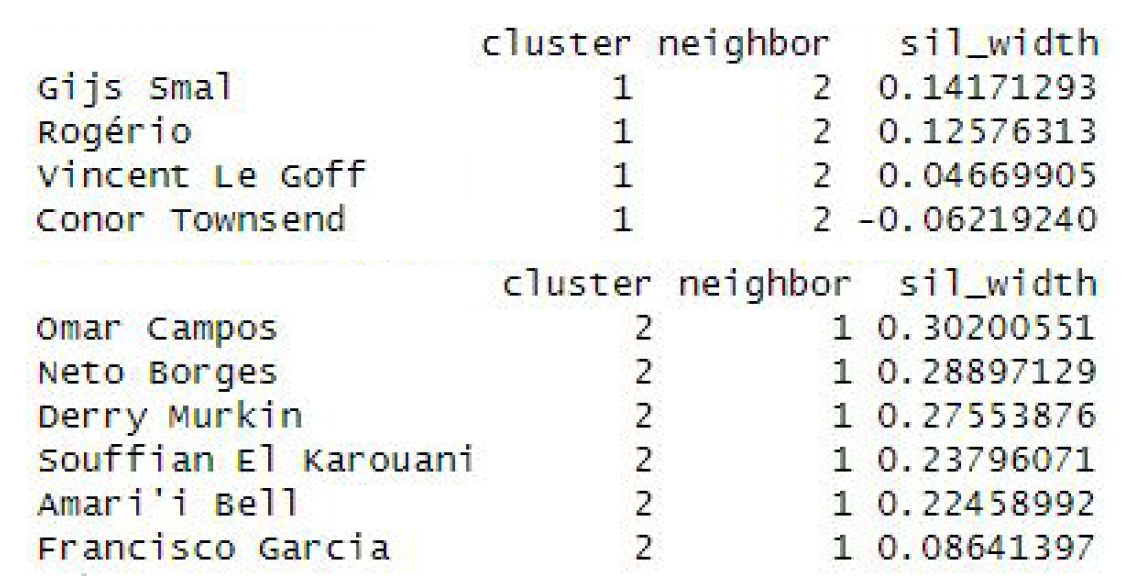
Santi Comesaña

Sergio Camello
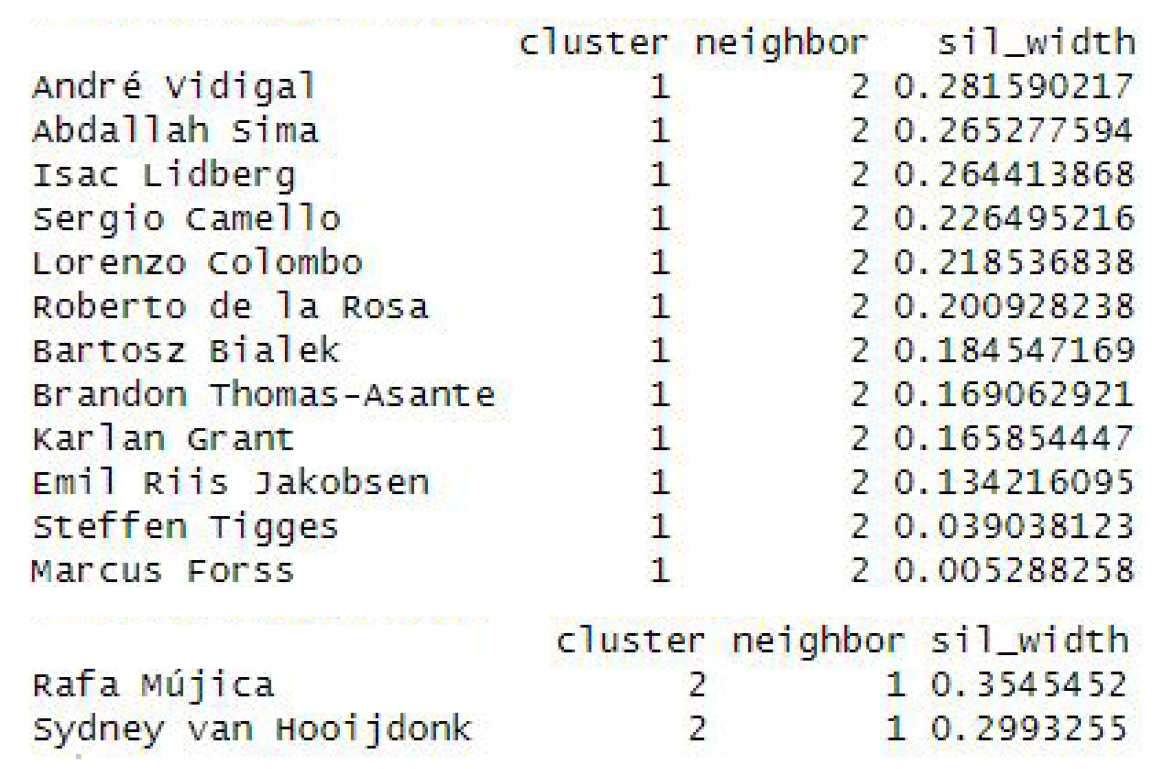
Radamel Falcao
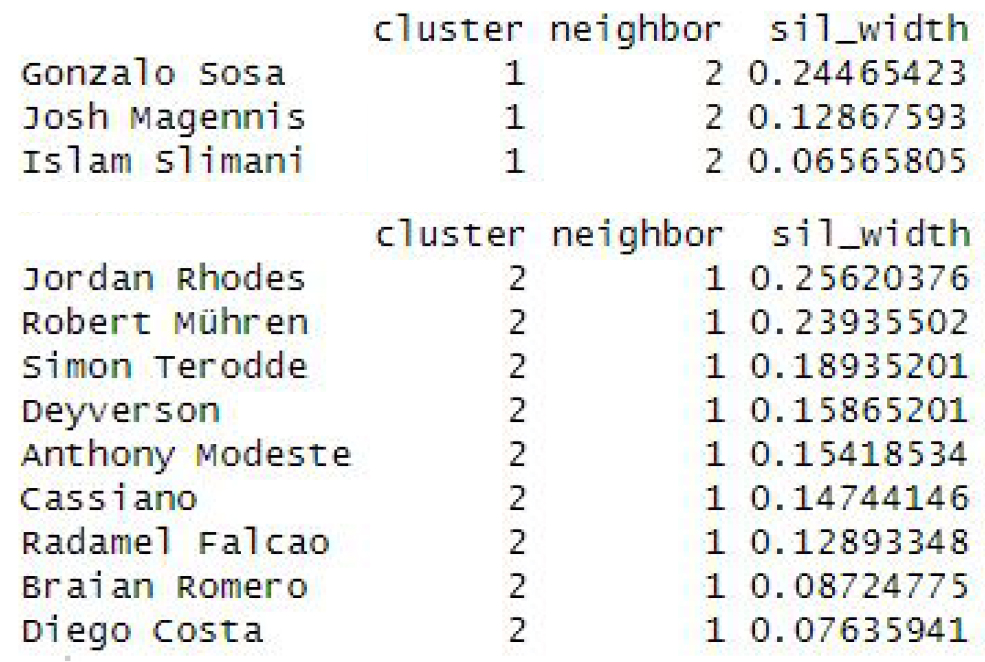
Source: Own elaboration.
Scoring
This ranking will be the culmination of all the analysis processes we have carried out. We have made a selection of players based on the correlation factor, then we have divided that sample into clusters to further refine the similarity and now with this ranking we will get a list, which although not definitive, will be the basis for the final choice.
This process will be carried out with R and it will be the last step we will take to help us choose one player over another in order to make the best decision. This will allow us to have a classification of the sample based on certain metrics that we will have to choose depending on the type of player we want.
We apply values or weights to these metrics that we select, which, once normalised, will be added up and then converted into percentages in order to order our selection.
It is important to understand the concept of weights, as it is the fundamental part of this process because the importance we give to each variable we use will make our scoring one or the other.
But we must also consider the context in which we move. As we said before, Rayo Vallecano is a club with a very limited budget, and that’s why we have taken this fact in mind to make the final selection of the sample, discarding candidates with high correlation levels, but unfeasible for the club.
Well, to make this ranking we also have to take this fact in mind, so we are going to add the metric we have of the market value based on Transfermarkt to the metrics we choose to, in this case, penalise the more expensive players over the cheaper ones.
Roughly speaking, what we are going to do is study each player we want to select to see where they stand out, which will let us know which metrics we should give more importance to, because, in the end, what we are looking for from the beginning are players similar to a given player, therefore, we should base it on their strengths and weaknesses.

Scoring results of all players
Alejandro Catena

Florian Lejeune

Fran García
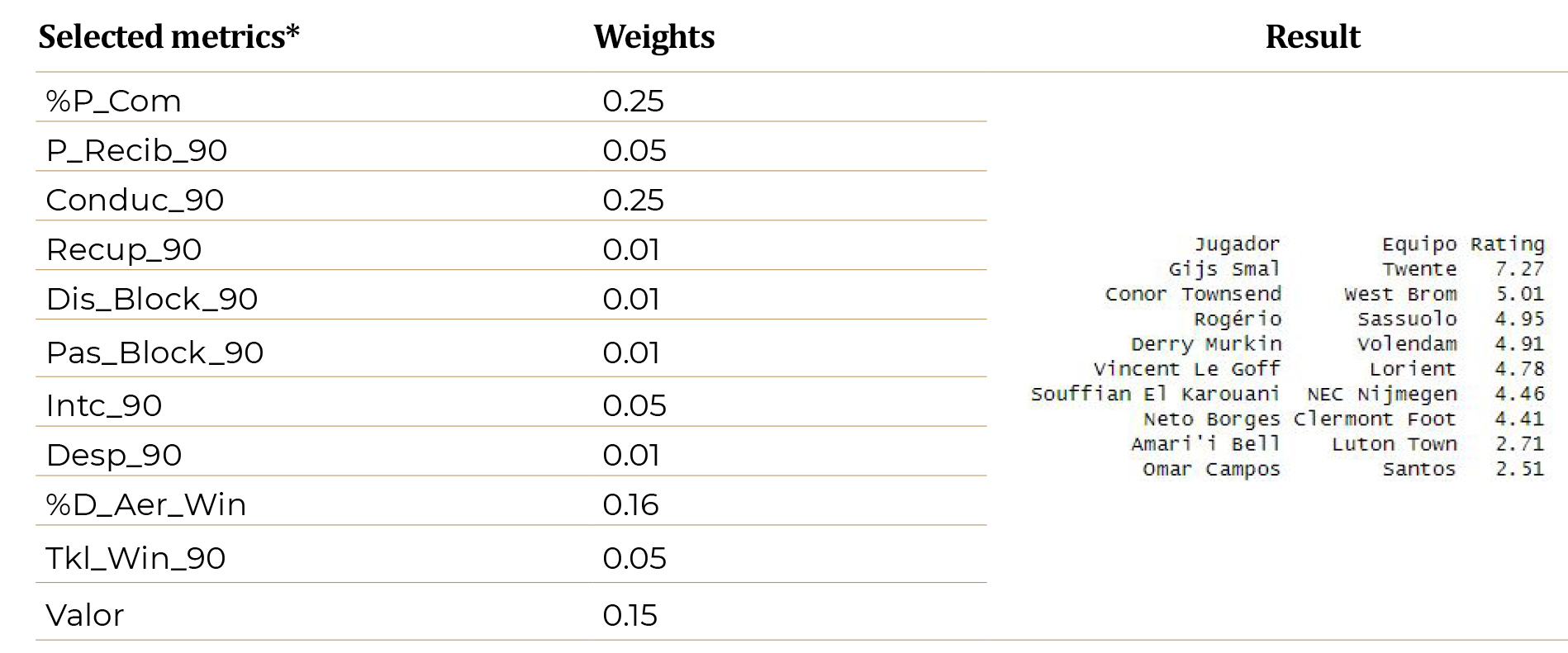
Santi Comesaña

Sergio Camello

Radamel Falcao

Source: Own elaboration.
* For further information on metric nomenclatures, see glossary at the end of the document.
VISUALISATIONS
In the previous step we have managed to separate the players into clusters and, in addition, we have obtained the crucial part of the process, on the one hand, the correlation index and, on the other hand, the scoring. These are all numbers, but now, to finish fitting everything together, it is necessary to translate all the information collected into different visualisations to analyse the selected players in depth with respect to our model.
These visualisations will help us to better understand all the extracted data and I will use specific tools such as Tableau and Power BI, as well as Python and R for this purpose.

It is important to note that we will not see big differences between any player precisely because we have made a selection based on the correlation factor, so we have ensured that all these players are similar and now we will finish refining the search.
The first step will be to make a summary presentation where we will show a funnel chart generated in Power BI with the percentage of correlation or similarity of the player to be replaced with the rest of the players, as well as the chart resulting from clustering in R (there will also be a small infographic with the players separated into their clusters).
When looking at the groups that R has generated in the clustering, we should not fall into the trap of believing that the players with the highest correlation index should be in the same cluster and the others in the next one.
If we remember, the clustering has been done using fewer metrics than in the dimensionality reduction, because some of them were removed as they had outliers that could distort the result of the search for these clusters, therefore, the result is also somewhat different.
After this introductory page, we see a table with a statistical summary by conditional colours, where, at a quick glance, we can see which players stand out in each metric… the darker the colour, the more they stand out in that metric, and vice versa.
This numerical information is important because it will help us to know the exact values of each metric for all players. In the charts we will not see these values reflected, since in these, what we will do is to position the players within a specific context.
The next step will be to generate different scatter plots where we will be able to observe all the players with respect to certain metrics, regardless of the cluster they belong to.
I find scatter charts very interesting, but always having in mind which variables we want to show. It is not simply a matter of showing one metric after another and, for that reason, I have always tried to give a cause and effect view, for example, I have compared the metric of passes received with that of control errors… Why? For an obvious reason… the more passes you receive, the more likely you are to make a mistake in ball control… cause and effect.
To finish the visualisations part, a comparison of several metrics that are interesting and have not been shown in previous charts will be displayed in a Lollipop (Tableau) chart. This type of charts, being also very visual, allow us to quickly compare all the players.
Finally, and as a conclusion, an infographic will be made with the results obtained in the scoring, as well as a final page with the three selected candidates where we will see their metrics compared with the player to be replaced by means of radar charts.



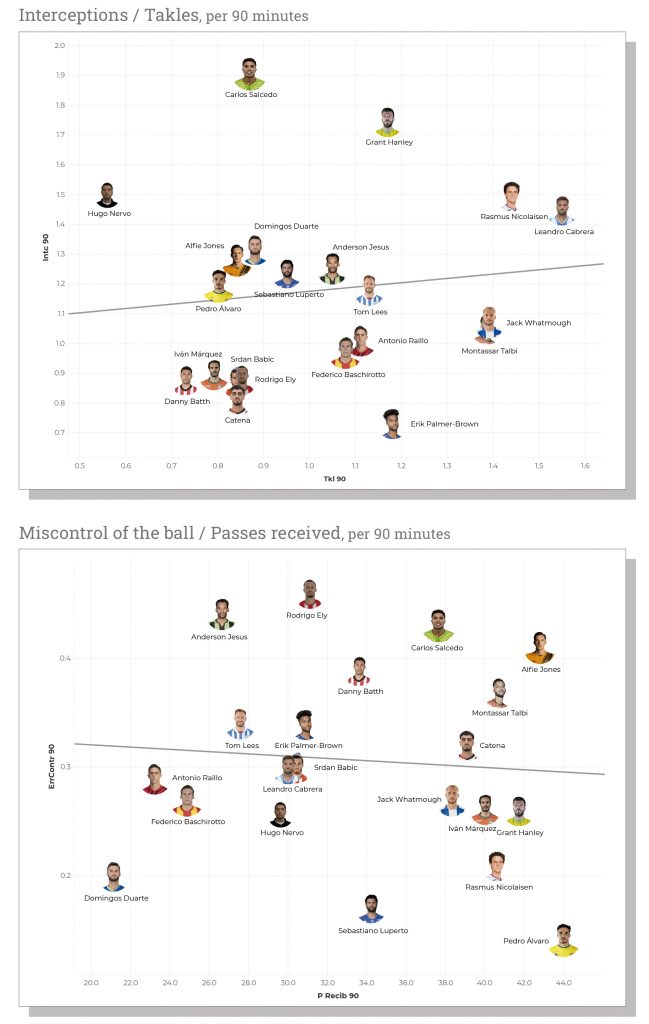


CONCLUSIONS
Making a global evaluation based on all the data that has been shown, on all the analysis processes carried out and in the economic context that was set (maximum 8 million market value), I consider that Rasmus Nicolaisen, Erik Palmer-Brown and Rodrigo Ely would be the three main candidates to replace Catena.
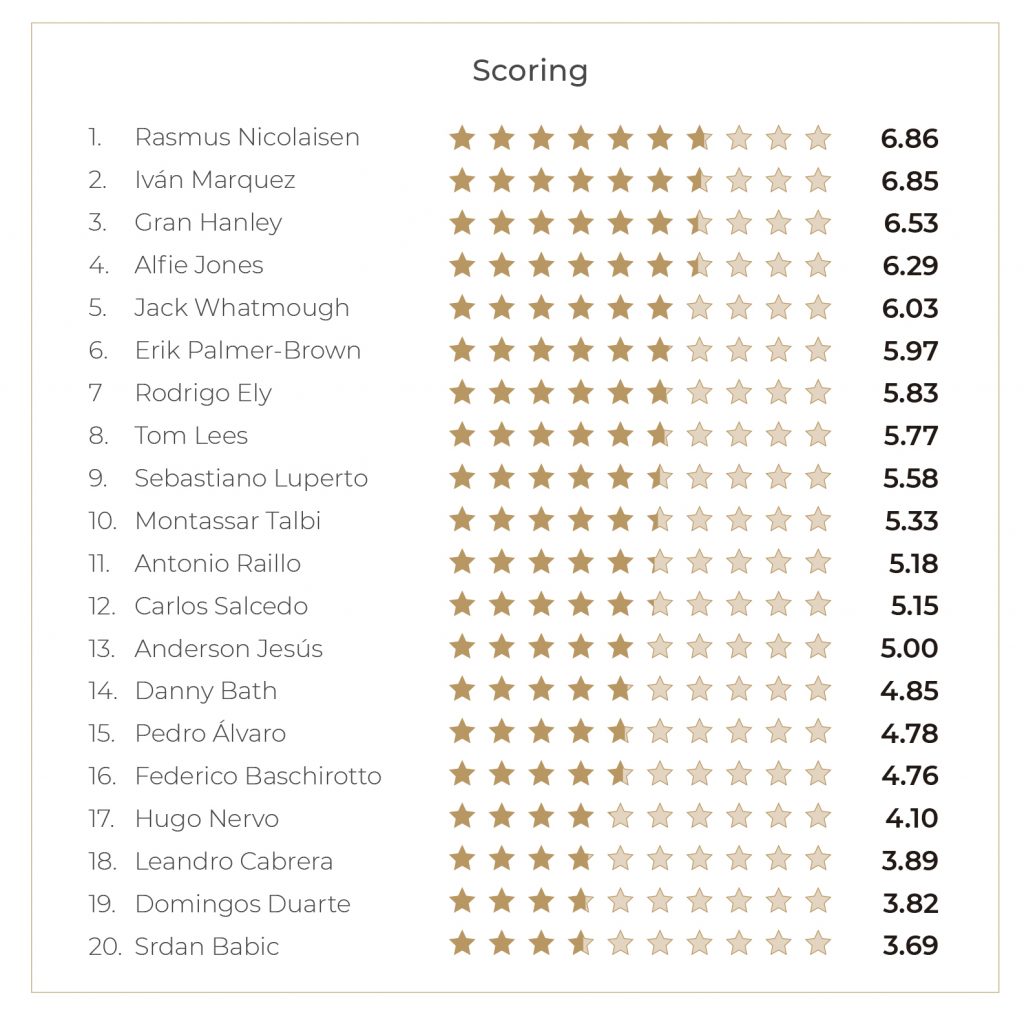
Ivan Marquez was originally among the top three candidates, but at the beginning of July he moved to FC Nürnberg as a free agent, making his signing unlikely and replacing him by Rodrigo Ely.
As can be seen in the scoring, Grant Hanley, Alfie Jones and Jack Whatmough appear ahead of two of the candidates.
Signing English players is always something to study in depth as they tend not to adapt easily, both because of the language and the change in style of play, therefore, even if they appear in the top positions, it will be preferable to opt for another option provided that there are other similar players.
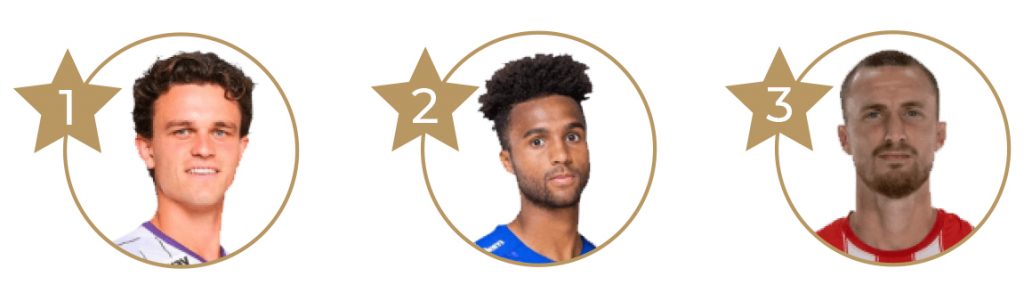
1. Rasmus Nicolaisen. Danish player who plays for Toulouse in Ligue 1. Similar in age to Catena, he excels in all defensive metrics and at first glance would be an optimal candidate, but he has the handicap of his price, having a high market value for a club like Rayo. Also playing against him is the fact that his club has won the Coupe de France and it might be difficult to think about a move to a team of a similar level.
2. Erik Palmer-Brown. American who plays for Troyes in Ligue 1. Very similar in recoveries, clearances, interceptions and in the percentage of aerial duels won, and superior in tackles and blocked passes. He is valued at 2.50 million, but his contract expires in 2024, so the club may be open to a possible transfer in this transfer window. It could be a market opportunity due to his team’s relegation to Ligue 2.
3. Rodrigo Ely. Brazilian with Italian passport who plays for Unión Deportiva Almería. He shines in clearances and in the percentages of aerial duels won and passes completed. He knows La Liga perfectly well so he would not need acclimatisation, he is valued at three million, but his contract ends in 2024.
I would mention Leandro Cabrera as a fourth option because, as with Erik Palmer-Brown, his team has been relegated to the second division and he could be another market opportunity because his contractual situation could be beneficial for a possible financial transfer or a loan.

Source of charts: Own elaboration inspired by StatsBomb / Rami Moghadam.





CONCLUSIONS
Making a global evaluation based on all the data that have been shown, on all the analysis processes carried out and in the economic context that was set (maximum 8 million market value), I consider that Pedro Álvaro, Yoann Salmier and Riccieli would be the three main candidates to replace Lejeune.
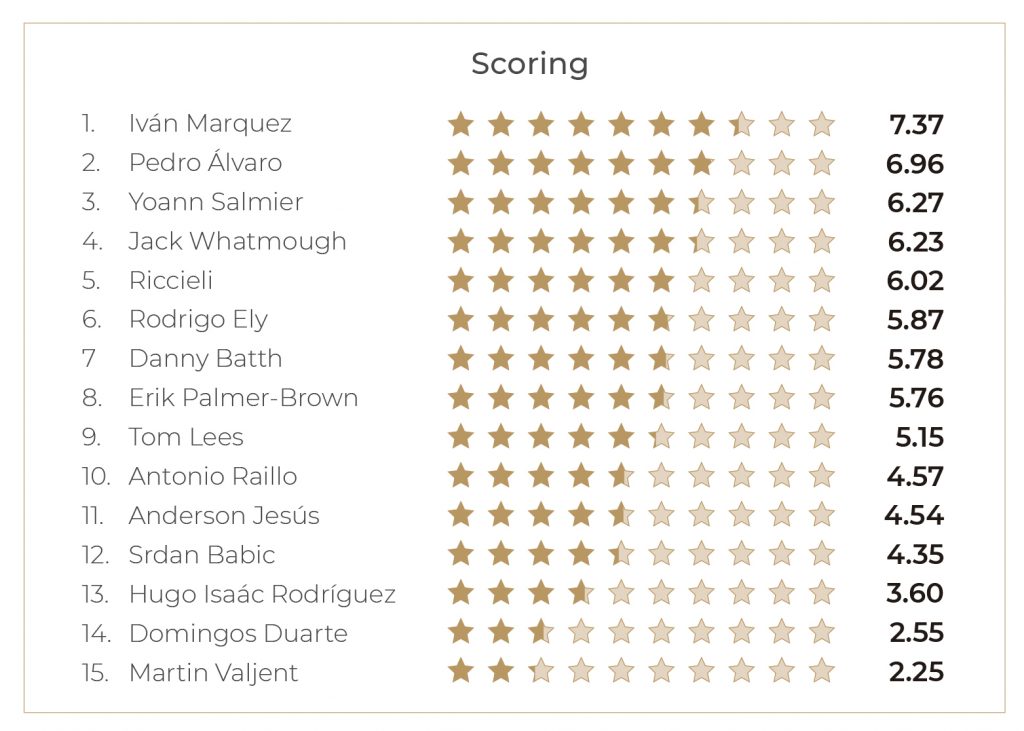
Iván Márquez and Jack Whatmough have been removed due to the circumstances described above.
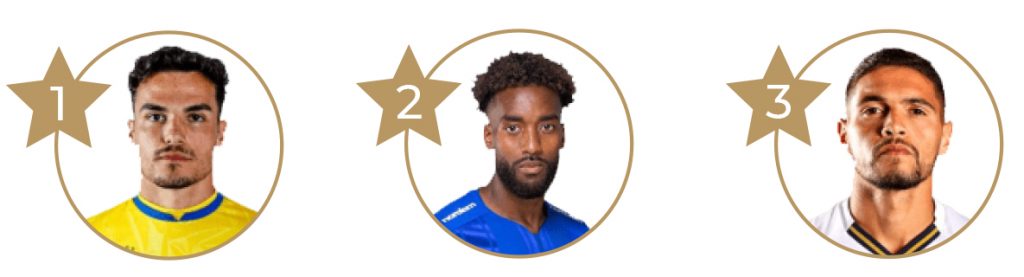
1. Pedro Álvaro. Young Portuguese player trained in Benfica’s youth academy and now plays for Estoril Praia in the Primeira Liga. Very similar in the percentages of aerial duels won and passes completed, as well as in clearances and superior in interceptions, blocked shots and passes received. His high similarity rate to Lejeune, his margin for improvement as a very young player and his affordable market value make Pedro Álvaro an optimal candidate.
2. Yoann Salmier. French player who plays for Troyes, partner at the back of Erik Palmer-Brown, who is among the players selected to replace Catena. He beats Lejeune in almost every metric and he is especially outstanding in interceptions, driving and recoveries.
3. Riccieli. 24 years old Brazilian who plays for Famalicão in the Primeira Liga. He shines in clearances, pass completion percentage and blocked passes and shots, but suffers from Lejeune in recoveries and driving.

Source of charts: Own elaboration inspired by StatsBomb / Rami Moghadam.



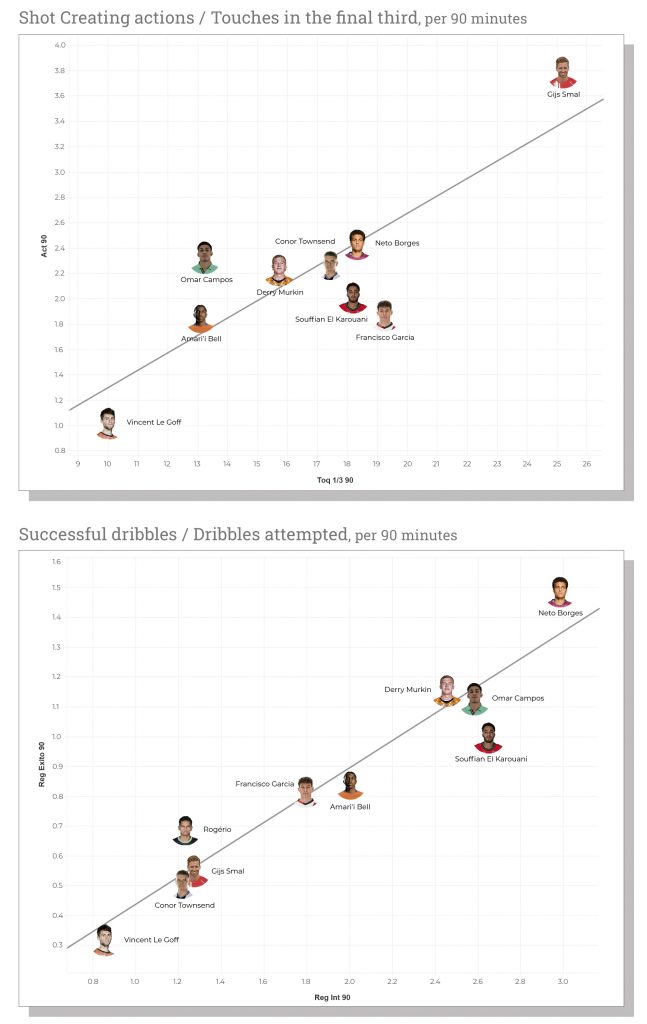

CONCLUSIONS
Making a global assessment based on all the data that has been shown, on all the analysis processes carried out and in the economic context that was set (maximum 8 million market value), I consider that Gijs Smal, Conor Townsend and Rogério would be the three main candidates to replace Fran García.

On this occasion I have kept Conor Townsend among the players chosen because the market for left-backs is smaller, there are not so many affordable options, so, although he is a British player, the fact that his market value is lower than many of the other players has been more important in his choice.

1. Gijs Smal. 26 years old Dutch player who plays for Twente in the Eredivisie. He is the best candidate, but his high market value (6 million) works against him. As for his metrics, they are very high in almost all of them, especially in passes and touches in the last quarter, progressive passes, passes to the penalty area or shot creation actions. He only suffers somewhat with respect to Fran García in his carries in the final third.
2. Conor Townsend. English player who plays for West Brom in the Championship. Similar to Fran Garcia in many of his metrics, he also suffers in relation to him especially in carries in the final third. His handicap, as mentioned before, could be a possible difficult adaptation to the change of league and language.
3. Rogério. 25 years old Brazilian who plays for Sassuolo. He is similar in metrics to Conor Townsend, and, like him, struggles with Fran Garcia in carries final third, although he outperforms them both in progressive passing and passes into the penalty area.
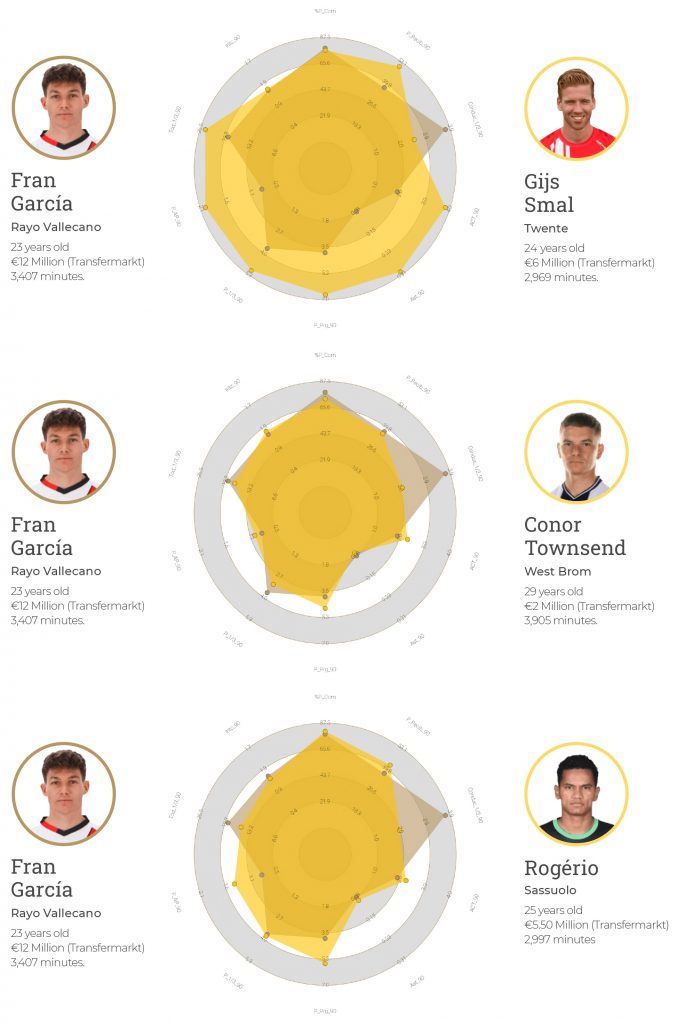
Source of charts: Own elaboration inspired by StatsBomb / Rami Moghadam.
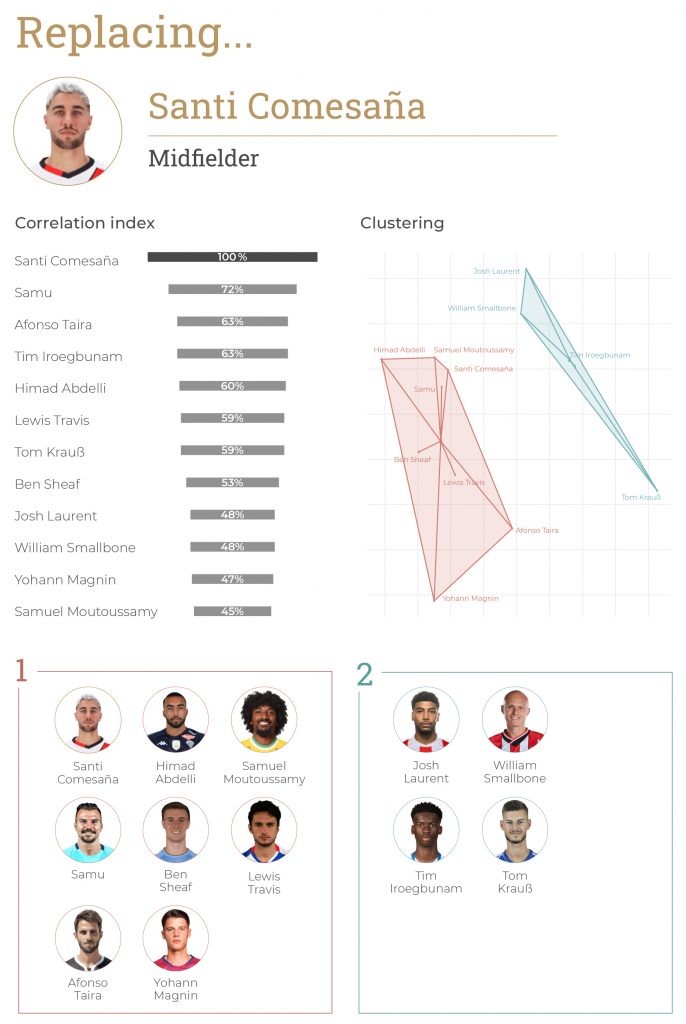
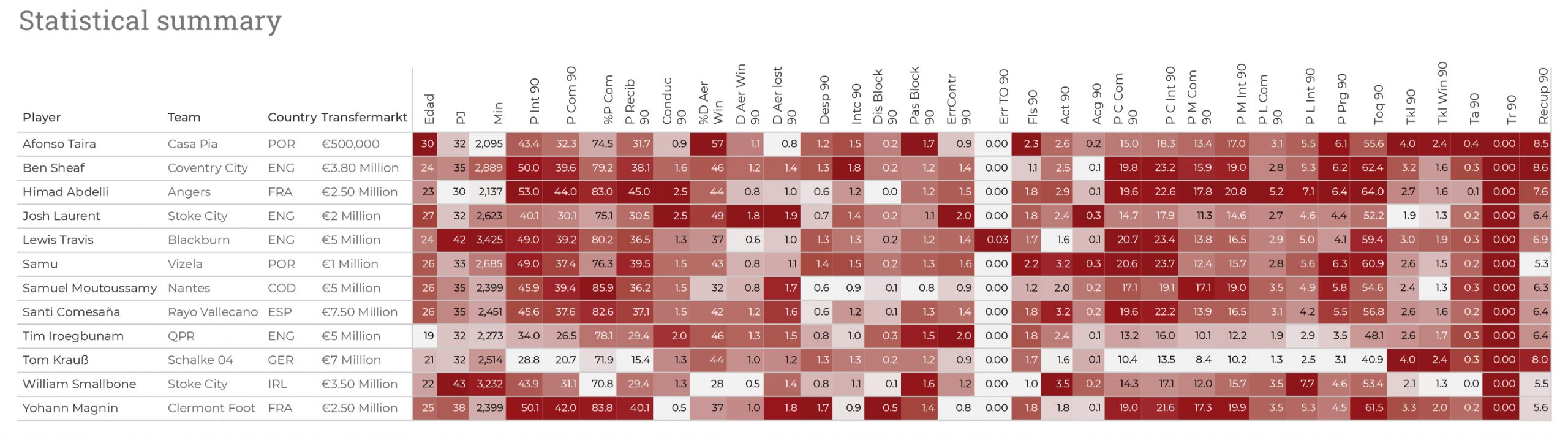



CONCLUSIONS
Making a global evaluation based on all the data that have been shown, on all the analysis processes carried out and in the economic context that was set (maximum 8 million market value), I consider that Himad Abdelli, Samu and Yohann Magnin would be the three main candidates to replace Comesaña.

Ben Sheaf has been excluded because he is the player with the highest market value in the top four and he is also British, so I think Yohann Magnin may be a more realistic target.
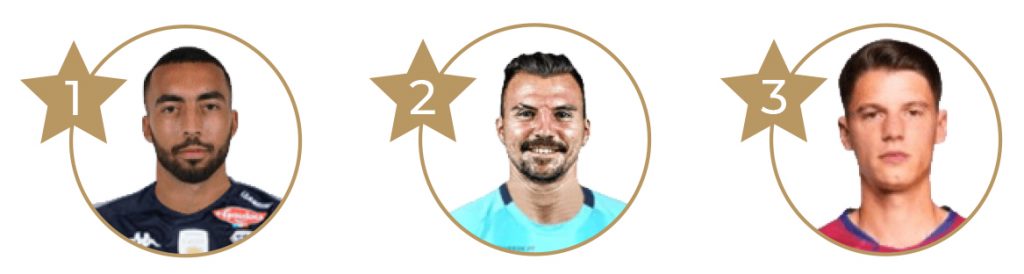
1. Himad Abdelli. 23 years old French player who plays for Angers, outperforms Comesaña in almost every metric, especially in touches, progressive passes and long and medium completed passes. He could be a market opportunity due to his team’s relegation to Ligue 2.
2. Samu. Portuguese player who plays for Vizela in the Primeira Liga. Unlike Himad Abdelli, Samu shines more in completed short passes than in long or medium ones, so we can deduce that his football is more about touch, in fact, his metric in touches is very high. He suffers with respect to Comesaña in recoveries.
3. Yohann Magnin. 25 years old Frenchman who plays for Clermont in Ligue 1. Great metrics of touches, medium passes completed and pass completed percentage. Suffers from Comesaña in shot creation, interceptions and progressive passing.

Source of charts: Own elaboration inspired by StatsBomb / Rami Moghadam.
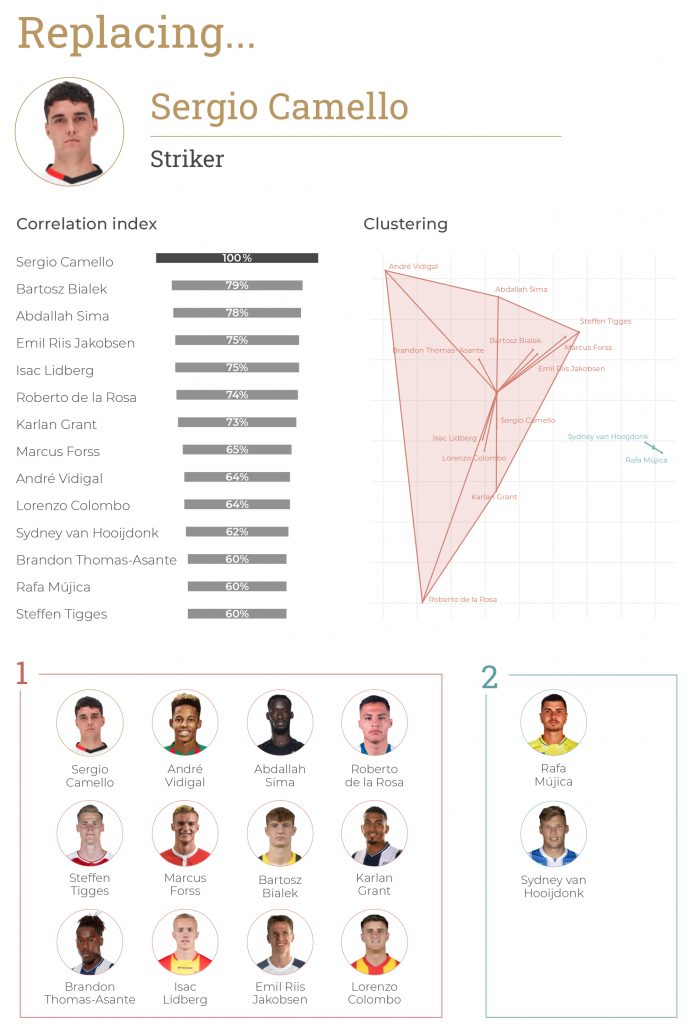
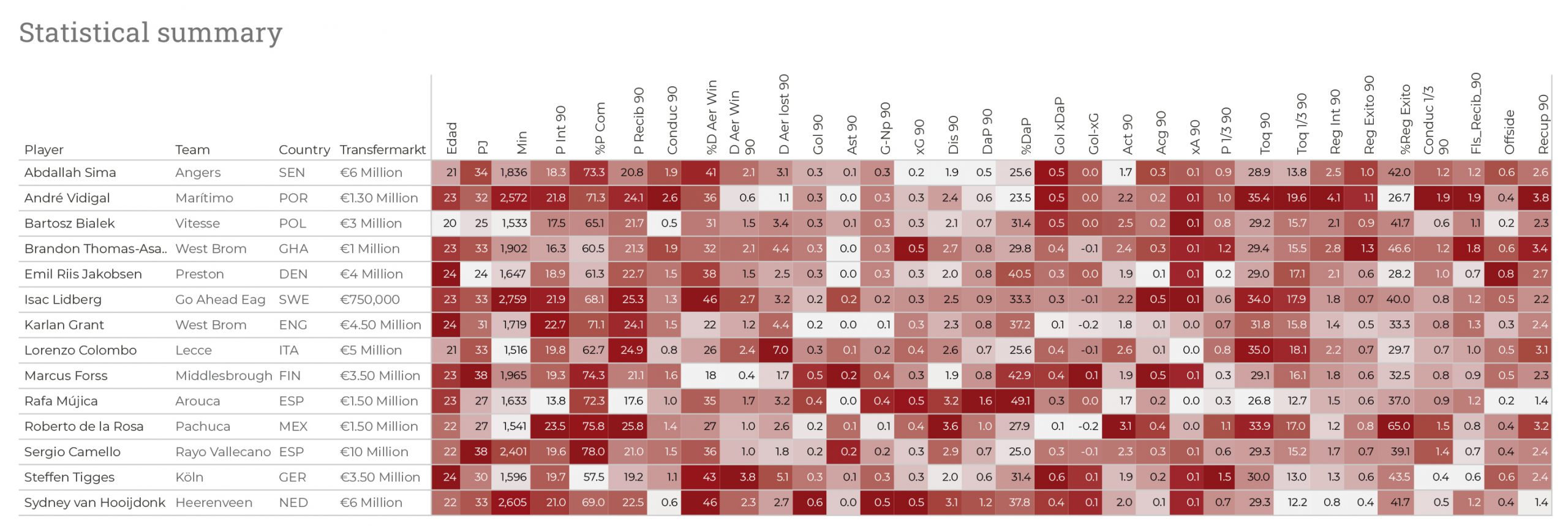



CONCLUSIONS
Making a global evaluation based on all the data that has been shown, on all the analysis processes carried out and in the economic context that was set (maximum 8 million market value), I consider that Marcus Forss, Isac Lidberg and Roberto de la Rosa would be the three main candidates to replace Sergio Camello.

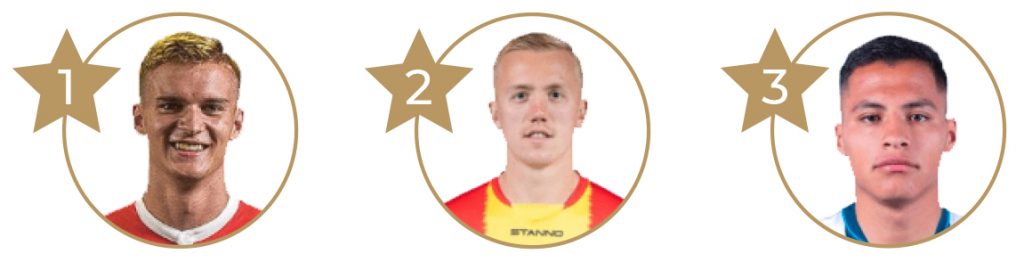
1. Marcus Forss. 23 years old Finnish player who plays for Middlesbrough in the Championship. He beats Camello by far in goals per 90 minutes, and also in shots on target. On the other hand, Camello beats him in shot creation and in the percentage of successful dribbles.
2. Isac Lidberg. 23 years old Swedish player who plays for Go Ahead Eagles in the Eredivisie. Similar goals per 90 minutes metric to Camello, outperforms him in shots on target, percentage of shots on target, passes received, xG and touches in the final third and has a lower assists per ninety minutes metric.
3. Roberto de la Rosa. 22 years old Mexican who plays for Pachuca in Liga MX. He outperforms Camello in almost every metric except goals per 90 minutes and assists per ninety minutes. He shines in shots on target, passes received and percentage of successful dribbles.

Source of charts: Own elaboration inspired by StatsBomb / Rami Moghadam.

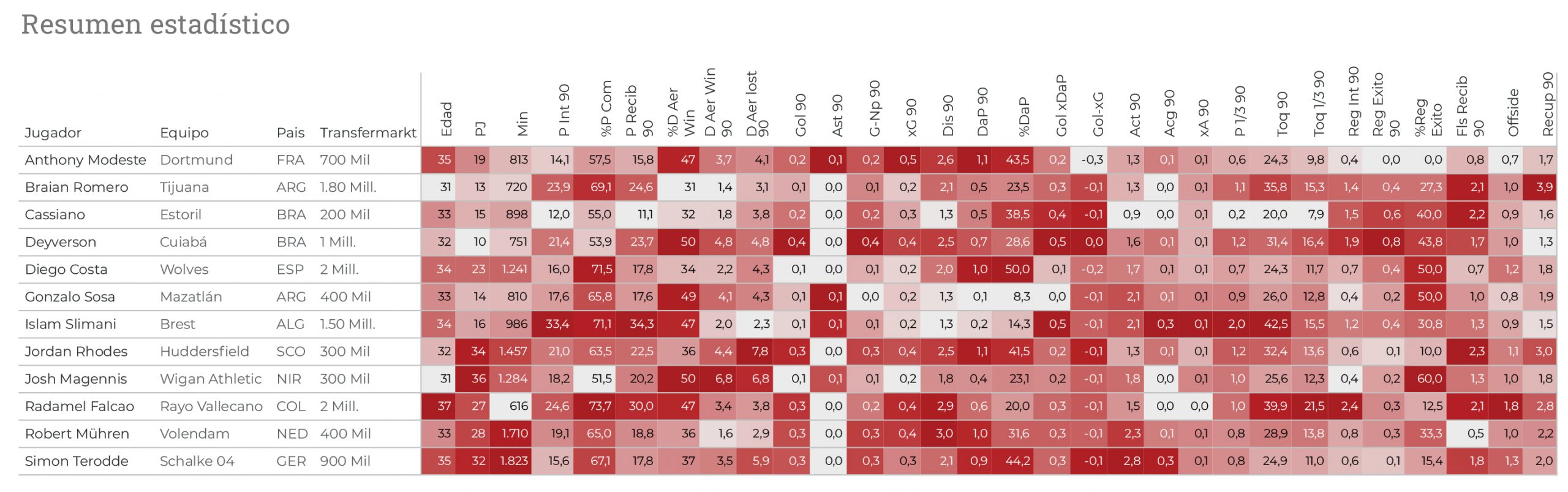


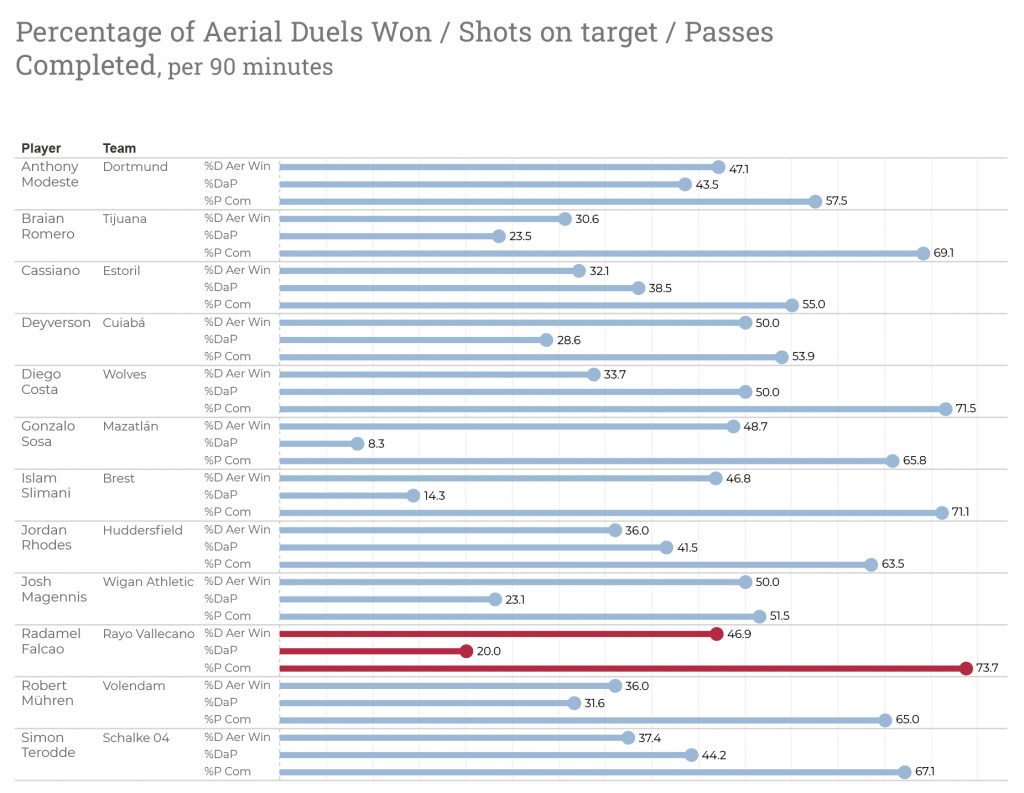
CONCLUSIONS
Making a global evaluation based on all the data that have been shown, on all the analysis processes carried out and in the economic context that was set (maximum 8 million market value), I consider that Deyverson, Jordan Rhodes and Robert Mühren would be the three main candidates to replace Radamel Falcao.


1. Deyverson. 32 years old Brazilian player who plays for Cuiabá in the Brazilian Serie A. Similar in assists and shot creation, he beats Falcao in goals per ninety minutes, shots on target and the percentage of these shots on target, while he suffers in the comparison in passes received, touches in the final third and recoveries. With experience in La Liga after playing for Levante, Getafe and Alavés and still at a very usable age, he is a prime candidate.
2. Jordan Rhodes. 32 years old English player who plays for Huddersfield in the Championship. Slightly better in goals per 90 minutes and, above all, in shots on target and the percentage of these. Like Deyverson, he suffers in the comparison in passes received and touches in the final third, as well as in xG. His handicap, adaptation, his strong point, his accessible market value (€300,000).
3. Robert Mühren. 33 years old Dutchman who plays for Volendam in the Eredivisie. He shines in shots on target and shot creation and, as with the two previous players, suffers in comparison to Falcao in passes received and touches in the final third, as well as in recoveries.

Source of charts: Own elaboration inspired by StatsBomb / Rami Moghadam.
GLOSSARY AND REFERENCES
For the correct understanding of the terms of all the metrics that appear throughout the document, a glossary is attached with the nomenclature that has been finally used after having done the whole process of cleaning the data downloaded from the fbref website.
Glossary

